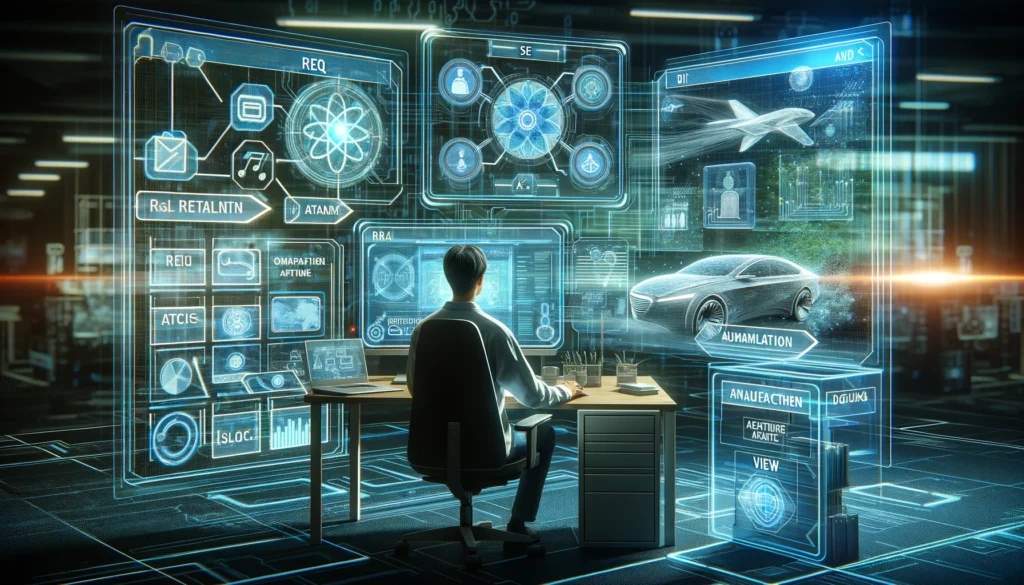
In the landscape of rapid technological advancement, the intersection of Artificial Intelligence (AI) and job roles is a topic of intense discussion. As Salman Khan insightfully points out in his new book “Brave New Words,” people aren’t replaced by AI but by individuals who leverage AI more productively. This perspective, while intriguing, calls for a deeper exploration of how AI impacts various job roles in today’s market.
Understanding AI’s Impact on Systems Engineering
One fascinating approach to gauge AI’s influence on jobs is proposed by There’s an AI for That. The website offers an ‘Impact Index’ for various job roles, including Software Engineer, Systems Engineer, Mechanical Engineer, and thousands of others. This index is calculated based on the number of AI applications that support tasks related to these roles. While specific and limited, this approach provides a more concrete perspective than speculative opinions like ‘I believe AI will never …’ or ‘Everybody will loose their jobs’ that are vague beliefs at best and sometimes even cause unnecessary alarm.
Breaking Down Job Roles into Tasks
To truly understand AI’s impact on jobs, it’s essential to break them down into their constituent tasks. For each task, we must examine whether AI can already support it effectively, whether AI needs refinement or regulation, or whether AI is currently incapable of supporting the task.
Sal Khan’s book serves as a real eye-opener in this context illuminating what can be achieved in one year if you go all in. E. g., the one-on-one AI tutor called ‘Khanmigo’ initially gone live along GPT-4 shall not give the final answers right away, but instead guide the student step by step (a so-called Socratic tutor). It can summarize the learning process and give valuable feedback to the teacher incl. recommendations. It can even identify weak spots the teacher might wish to focus on. And it assists the teacher in creating lesson plans saving a lot of time and freeing resources.
Drawing inspiration from this work, one could investigate systems engineering in a similar vein and develop an ‘MBSEamigo’ analogous to ‘Khanmigo’. The core idea here is to redefine roles based on tasks. In situations where role definitions vary, the strategy would be to identify common denominators and refine roles down to the tasks.
For instance, consider the role of a systems engineer. This job can be broken down into approximately 1,000 tasks. Each task can then be assessed for AI impact. Here’s a glimpse into some typical tasks and their AI impact:
- Running Coach: AI Impact: 100% | AIs: 12
- Codebase Q&A: AI Impact: 95% | AIs: 9
- Problem Solving: AI Impact: 90% | AIs: 15
- Diagrams: AI Impact: 50% | AIs: 12
- Data Protection: AI Impact: 50% | AIs: 4
- Conversations with Clients: AI Impact: 50% | AIs: 1
- Audits: AI Impact: 5% | AIs: 1
- 3D Images: AI Impact: 5% | AIs: 22
A Closer Look at Key Tasks
Running Coach (AI Impact: 100%)
In systems engineering, optimizing processes and workflows is crucial. AI tools can act as running coaches, continuously analyzing and improving these processes with precision and speed. For example, AI can automate routine checks and suggest improvements, ensuring systems run smoothly and efficiently.
Codebase Q&A (AI Impact: 95%)
AI-driven tools like code analyzers and automated testing frameworks significantly enhance codebase management. They can identify bugs, suggest fixes, and predict potential issues before they become critical, thereby reducing downtime and increasing productivity.
Problem Solving (AI Impact: 90%)
AI excels in problem-solving by offering data-driven insights and predictive analytics. For systems engineers, this means quicker diagnosis of issues and more effective solutions. AI can simulate various scenarios to find the most optimal solutions, saving time and resources.
Applying Foundational Engineering Principles
Incorporating AI into systems engineering must align with foundational engineering principles. This means ensuring that AI tools are used to enhance precision, reliability, and efficiency. Systems engineers should focus on maintaining robustness and accuracy in their projects while leveraging AI to handle repetitive and data-intensive tasks.
To do this:
- Verification and Validation: Regularly test AI tools to ensure they produce reliable and accurate results.
- System Integration: Seamlessly integrate AI into existing systems without disrupting core functionalities.
- Continuous Improvement: Use AI for ongoing analysis and optimization of engineering processes.
- Documentation and Transparency: Keep thorough documentation of AI’s role and decisions in the engineering process for transparency and traceability.
The Future of Systems Engineering with AI
The journey of AI in systems engineering is just beginning. By continually refining AI tools and expanding their capabilities, we can create more efficient, innovative, and secure systems.
As highlighted in Engineering.com, AI has the potential to revolutionize engineering by automating complex tasks, predicting system behaviors, and providing advanced analytics. Currently, using tools requires understanding how to manipulate and connect various elements, which involves tedious tasks like moving the mouse around. This repetitive work, similar to past challenges in the CAD world, is expected to be minimized in the future.
SElive also identifies potential AI use cases. This integration not only boosts productivity but also fosters innovation by enabling engineers to focus on more strategic and creative aspects of system development. One notable example is the Technology Summarizer, which employs AI algorithms to analyze and condense vast amounts of technical documentation. This tool helps engineers quickly grasp essential information, stay updated with the latest advancements, and make informed decisions without being bogged down by extensive reading.
The upcoming book “AI Assisted MBSE with SysML by Doug Rosenberg, Tim Weilkiens (mbse4u.com))” explores the integration of Artificial Intelligence in Model-Based Systems Engineering (MBSE) using the Systems Modeling Language (SysML). It highlights how AI can automate and enhance various MBSE tasks and provides methodologies along a comprehensive, step-by-step design of a Scanning Electron Microscope.
Final Thoughts
The integration of AI in the job market isn’t about replacement but about redefining roles and tasks so AI can be leveraged for increased productivity. The key lies in understanding AI’s impact on individual tasks and adapting job roles accordingly. As AI continues to evolve, our approach to integrating it into our workflows must also adapt, ensuring we stay ahead in this dynamic field.
I am convinced that we will see a net job effect again as we have been seeing with automation. To the younger generations: experts are still needed. Do not let social media make you panic! Anybody can get anything out of ChatGPT, but only the expert speaks the sophisticated language of a discipline achieving much better results that also need to be curated by the very same expert.