#architecture #clarity #velocity #direction
In my last post Executive Summary: Strategic Data Science, I have summarized what Data Science is and what it consist of. Moreover, you need to deploy a strategy that helps you manage transformation to a data-driven business.
Today, you will see that a strategy for data science can be handled just like any data strategy. And if you already have a data strategy deployed, e.g. as part of your governance or architecture initiative, then you will see why and where it is affected.
As written in Executive Summary on EA Maturity, having a map knowing where you are and where you want to go to helps a lot in finding a way.
Maturity
If you are working with maturity models, you typically do this on a yearly basis. For chosen capabilities you identify current vs target maturity e.g. ranked from level 1 to 5.
The first thing you need to understand is that introducing data science for the first time reduces your overall maturity at once. Why is that?
Maturity is measured in terms of capabilities. And if you take a look into those capabilities you will find that you need to adapt them. There typically are a dozen or so like vision, objectives, people, processes, policies, master data management, business intelligence, big data analytics, data quality, data modeling, data asset planning, data integration, and metadata management.
I will pick only a few as examples to make things clear. Let’s pick vision, people, and technology.
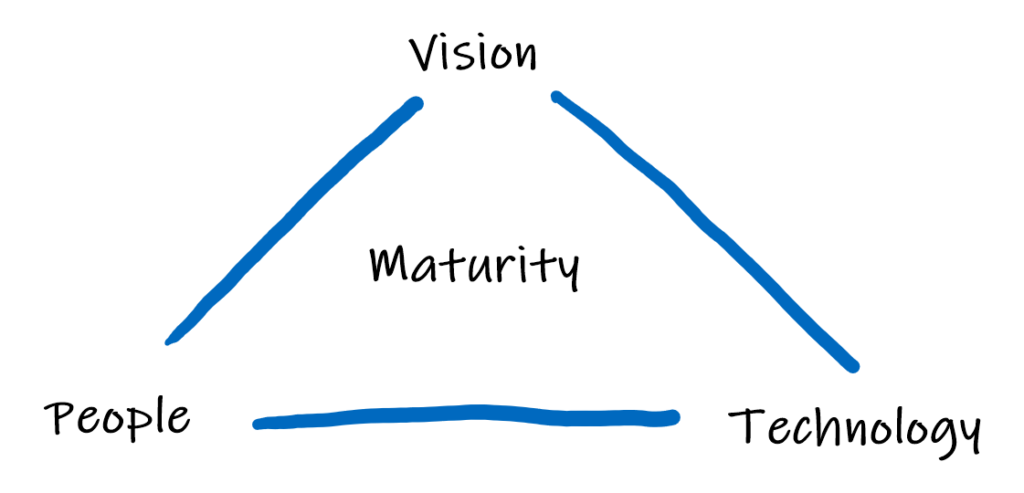
Vision
Say you have a vision like: “Providing customer care that is so satisfying, that every customer comes back to us with a smile”. That’s a very strong statement, but how about: “Keeping every customer satisfied by solving all problems before complaining”. Wow, even stronger. It is possible because Data Science allows you to predict what others can’t.
People
Probably, you already have a data architect. But, the classic data architect focuses on architecture, technology, and governance issues. This is OK, but you also need some data advisor focusing on unseen solutions for the business. Someone telling you to combine customer data with product usage data increasing your sales. And perhaps even telling you from which of your precious data you can create completely new data-driven products you can sell.
Technology
Probably, you also have an inventory telling you which data sources are used in your applications. Adding Data Science as rapidly growing discipline to the equation, you may find that you will have to revise your technology portfolio. It is rapidly growing and changing and, therefore, needs to be governed to a certain amount (freedom vs standardization).
Following list shows selected technologies that are most often used in Data Science (ranked from left to right).
- Programming Languages: SQL, Python, R
- Relational Databases: MySQL, MS SQL Server, PostgreSQL
- Big data platforms: Spark, Hive, MongoDB
- Spreadsheets, BI, Reporting: Excel, Power BI, QlikView
Moreover, there is a shift in who is actually using these technologies like Leadership, Finance, Sales, and Marketing. And more often without dedicated enterprise applications because data analysis is very dynamic and has a lot of try and error to it.
Conclusion
From these view capabilities out of a dozen+ it has become clear that Data Science Strategy easily fits into an overall Data Strategy. There is no need to reinvent the wheel. Instead, adapt your existing or favorite Data Strategy to incorparate Data Science.